
It’s the #1 most widely used data analysis and manipulation library for Python, and it’s not hard to see why. Pandas is one of the libraries powered by NumPy. Is it a surprise that a program that covers everything from sports to space can also help you manage and clean your data? Pandas It also confirmed the existence of gravitational waves, and it’s currently accelerating a variety of scientific studies and sports analytics. For example, NumPy enabled the Event Horizon Space Telescope to produce the first-ever image of black holes. Its high-level syntax allows programmers from any background or experience level to use its powerful data processing capabilities. It also offers a comprehensive toolbox of numerical computing tools like linear algebra routines, Fourier transforms, and more. Thanks to its speed and versatility, NumPy’s vectorization, indexing, and broadcasting concepts represent the de facto standard for array computing however, NumPy really shines when working with multi-dimensional arrays. In addition to serving as the foundation for other powerful libraries, NumPy has a number of qualities that make it indispensable for Python for data analysis. It’s also a fundamental library for the data science ecosystem because many of the most popular Python libraries like Pandas and Matplotlib are built on top of NumPy. NumPy is a fast and easy-to-use open-source scientific computing Python library. Here at Dataquest, we know the struggle, so we’re happy to share our top 15 picks for the most helpful Python libraries for data cleaning. The cleaner and more organized your data is, the faster, easier, and more efficient everything will be. There is no doubt that cleaning and preparing data is as tedious and painstaking as it is important. Messy data is useless data, which is why data scientists spend a majority of their time making sense of all the nonsense.
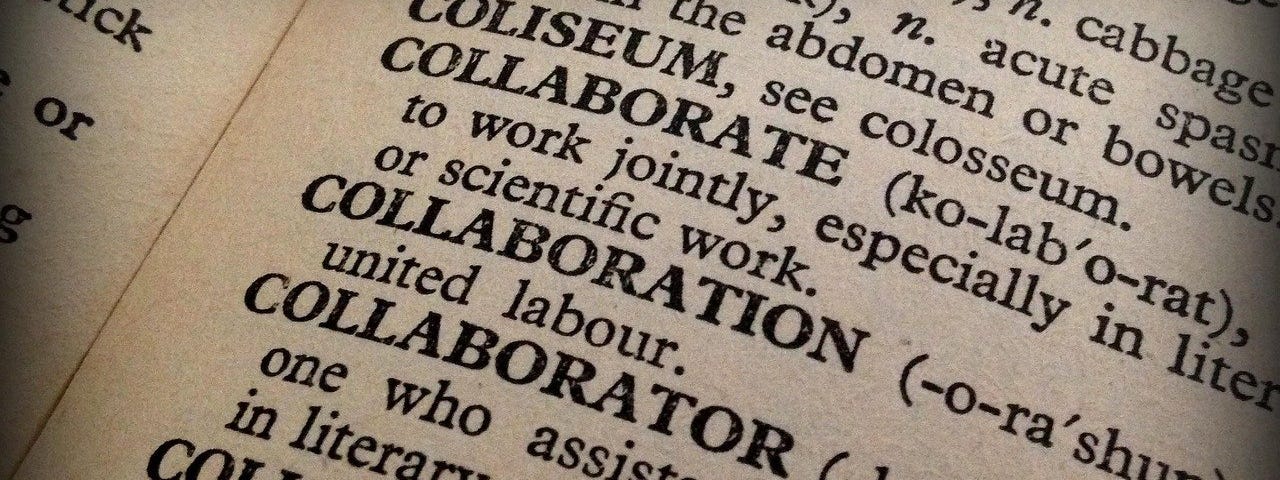
Especially when data comes from different sources, each one will have its own set of quirks, challenges, and irregularities.

Unfortunately, data is invariably going to have certain inconsistencies, missing inputs, irrelevant information, duplicate information, or downright errors there’s no getting around that. For many data workers, the cleaning and preparation of data is also their least favorite part of their job, so they spend the other 20-30% of their time complaining about it. Most surveys indicate that data scientists and data analysts spend 70-80% of their time cleaning and preparing data for analysis. Follow the steps on the page which asks you basic information about the project and your role.SeptemMost Helpful Python Libraries for Data Cleaning in 2021 This will allow you to get important keys to connect to the Twitter API. Step 2: Creating Projects & Apps within the Developer PortalĪfter setting up your developer account, create a new app and project in the developer portal.

The signup process is pretty straightforward, it might take a few minutes to complete the process but you should be all set after that. Setting up your Twitter Environment in 3 Steps Step 1: Setting up your Twitter Developer Accountįirst you have to sign up for a twitter developer account here: Here’s a step-by-step guide on how you can set up your account and generate a Twitter API.
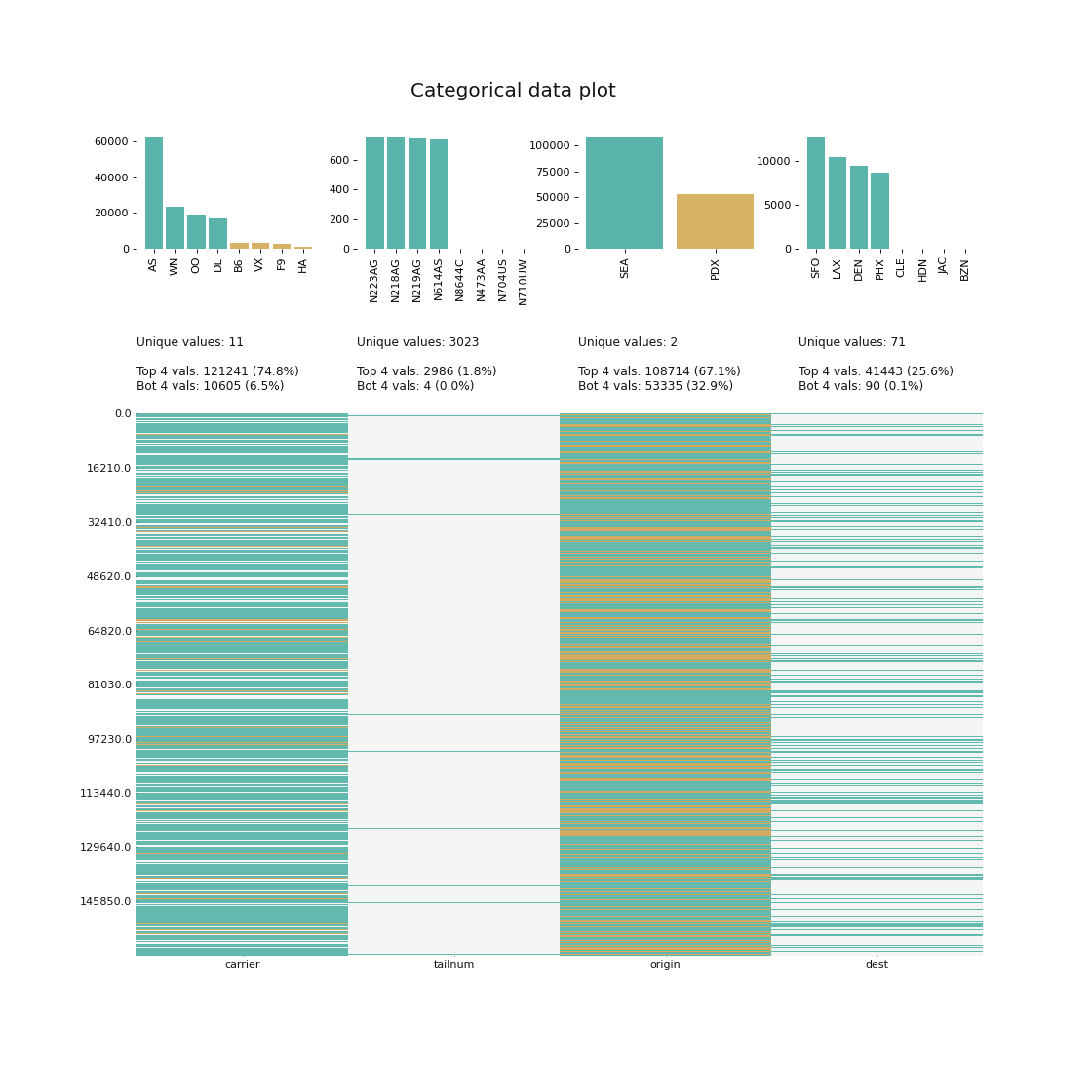
The first thing that you need to do before you can start collecting public tweets is to setup your Twitter Developer Account. If you’re passionate about Natural Language Processing or you want to collect and build your own data that you can use for your personal projects or analysis, Twitter is one of the best social media platforms where you can get huge text data.
